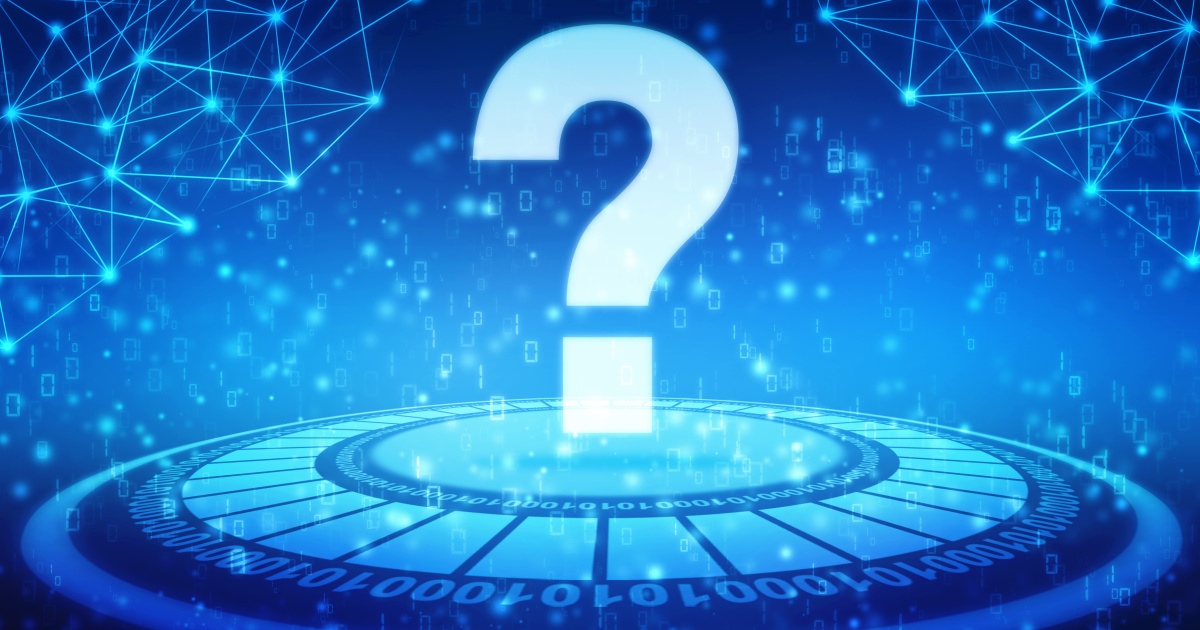
In today's world of search, people expect direct, accurate answers to their questions. Additionally, enterprises want detailed insight into their customers’ and employees’ information needs. Yet, despite their best efforts to provide quick, impactful responses – and the significant investments already made over multiple years – most enterprises struggle to quickly and accurately respond to customer and employee questions. They also fail to provide the precise information end users really want. This is primarily due to poorly performing keyword search engines, chatbots, and the understaffed call centers that must deal with all the inquiries that could not be addressed with self-service methods.
Simple keyword searches are unable to interpret user intent. Recently, vendors have been touting generative artificial intelligence (GenAI) as the tool to address these issues but, despite the intriguing promise and hype, GenAI alone cannot provide accurate answers reliably. Left unchecked, this combination of poorly performing keyword searches and chatbots and the unreliability of generative AI often leads to user frustration with the enterprise and wasted effort by internal content management teams. Rather than conventional keyword search engines that often return links to out-of-context information or generative AI that provides inaccurate information, what all stakeholders really need is a way to discover relevant and accurately summarized information to full questions instantly.
The underlying cause for the difficulty in simply answering end users’ questions is that 80% of enterprise data is unstructured (i.e., free form text documents, such as written content on web pages, word processing documents, surveys, contact center information, etc.), which makes it extremely difficult to identify and retrieve the information needed. Traditional search tools use keyword or token-based methods, which can’t interpret the intent or context behind the words in a question.
Although some tools are starting to advertise the addition of artificial intelligence elements, they are generally not easy for business owners to use directly without a lot of expensive IT or technical assistance. Likewise, most chatbots are built on traditional, rules-based approaches, which fall short of answering most user questions. According to Gartner, though 90% of enterprises have invested in chatbots, only 4% are actually using them efficiently. Research also suggests that 53% of chatbot projects were discontinued after 15 months – an extremely high failure rate.
To address this issue, vendors began promoting generative AI bolt-ons to their products as the solution. Early adopters have learned the painful lesson that transitioning the seemingly limitless possibilities of generative AI into a tool that reliably provides accurate information is not as easy as hoped. As a result, many organizations have started to move more cautiously to ensure that they understand all the implications of a generative AI effort and have tried several approaches to address the challenges of unstructured data, including:
- Adding structure to unstructured data with taxonomies and ontologies,
- Leveraging large language models (LLMs) and deep learning,
- Implementing Symbolic AI, and
- Applying quick fixes to generative AI issues.
However, to date, none of these approaches have substantially improved users’ ability to quickly find answers. This has led more and more customer support organizations and content creators to look for alternative approaches.
Why AI-enabled Answer Engines are the Next Generation of Search
To address these lingering challenges, enterprises are applying “answer engine” technology to bridge the gap. These next-generation solutions enable users to ask complete questions as if they were asking a subject matter expert and get the correct answer regardless of how they phrase the question. They also allow business users and SMEs to leverage AI technologies directly without relying on technical teams for support. Under the hood, the inherent capabilities of these answer engines include important features such as:
- Ability to significantly focus the input to generative AI: substantially limiting and focusing the input into large language models to eliminate hallucinations and ensure accurate and trusted answers.
- Insights into content users want to see: analytics reveal what users are really looking for, especially when they ask full-sentence questions suitable for answer engines.
- Natural Language Understanding (NLU): a broad set of cognitive techniques that understand the nuances of language, including domain-specific terms. It also understands the intent of words and phrases (e.g., questions starting with “who”, “when”, “where”, etc.) to understand that the user may be looking for data on a specific name, location, timeframe, or measure rather than a direct token match.
- Query Resiliency: provides consistent results no matter how various individual users phrase the question.
- Multi-level Explainability: contrary to most “black-box” AI tools, answer engines provide users with the capability to see the various strategies and scores used to find each result, assuring users’ confidence while also allowing them to tune the platform to incorporate new products, focus areas, and terminology.
- Easy Useability and Manageability: via a no-code platform and by eliminating the need for labeling/annotating or model training.
While time-to-answer is always a key consideration to driving user satisfaction, accuracy and trust in the results remain paramount. After all, there is no point in getting a fast but inaccurate answer. While every enterprise would suffer reputational risk from inaccurate information, some industries like banking and pharmaceutical have the additional burden of substantial regulatory requirements with respect to the answers they provide to end users.
Is ChatGPT an Answer Engine?
ChatGPT has shown the world what is possible in search. Although it has made great strides, and could be suitable for many consumer search applications, ChatGPT is not an answer engine because it too often returns unacceptable levels of inaccurate information and does not provide any explanation as to how it generated a response. Enterprises must provide both trusted answers and transparency and they simply cannot afford to rely on tools like ChatGPT… not yet, anyway.
Repeated customer surveys demonstrate that it is imperative for all enterprises to reconsider how they can be more effective in addressing users' questions. Many enterprises seem to have gotten the message regarding the importance of delivering timely and accurate answers and the impact on end user experience and consumer trust in their brand. Internal employees benefit from this capability as well. Gaining insight into the detailed and specific information users need will help guide the content team to develop relevant answers more efficiently.
Despite the availability of new technologies and approaches like answer engines, the reality is this will take time and a sustained effort from enterprise leaders to fully implement the changes that are necessary to achieve these goals. Nevertheless, the benefits to both internal and external will be well worth the effort as the alternatives simply are too risky to ignore.
About the Author: John Reuter is the Chief Strategy Officer at Kyndi, a global provider of the Kyndi Platform for the Natural-Language-Enabled Enterprise, an AI-powered platform that empowers people to do their most meaningful work. Follow Kyndi on LinkedIn and Twitter.
Edited by
Erik Linask