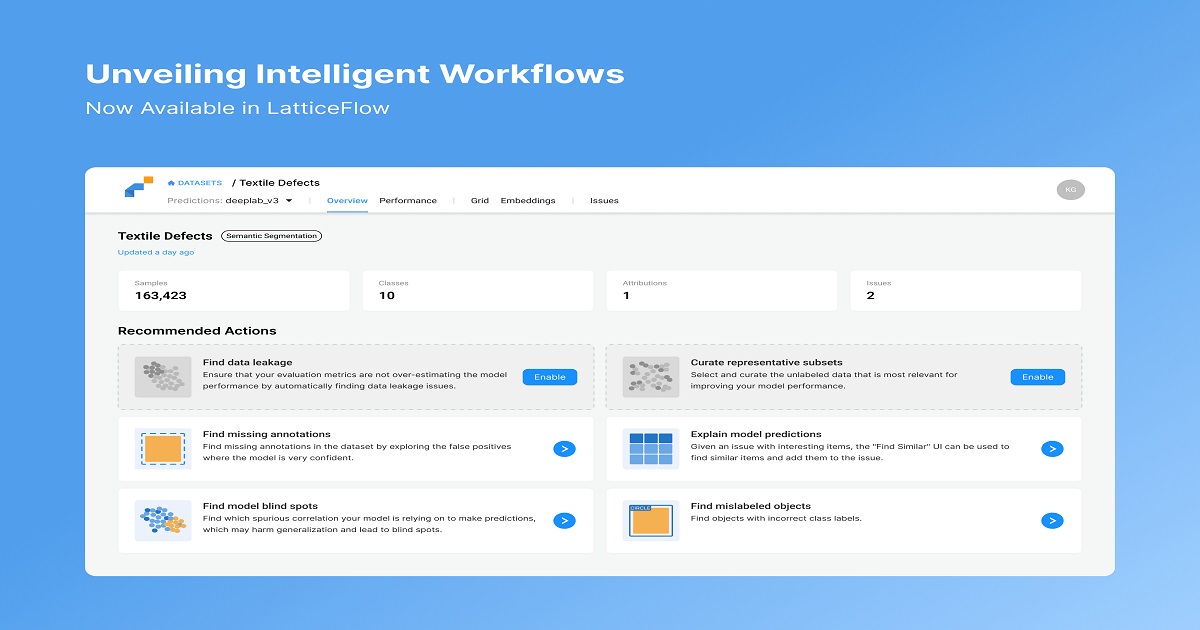
At least 35% of businesses have fully adopted AI, according to an IBM report. As AI becomes an integral component of business operations, there comes the challenge of effectively incorporating high-performing AI models into practical applications.
The successful deployment of AI models requires a commitment to automation and a systematic methodology for identifying and addressing potential blind spots. The complexity of managing vast amounts of data necessitates streamlined processes that can handle the intricacies of model integration, ensuring that AI solutions not only function optimally but also align seamlessly with the broader operational framework of the business.
Automation is pivotal for preparing AI models for production, streamlining deployment and reducing human error. A methodical approach emphasizes meticulous planning, testing and validation to identify potential blind spots that could undermine the reliability of deployed AI models. This involves a thorough evaluation of model performance in diverse scenarios to ensure excellence in controlled environments and robustness in dynamic real-world applications.
To provide that thorough evaluation, LatticeFlow has announced Intelligent Workflows.
LatticeFlow, an innovator in AI and machine learning (ML), is dedicated to fostering responsible AI development and deployment. LatticeFlow addresses model and data challenges through automated and intelligent workflows to help organizations unlock the full potential of AI across its lifecycle.
Intelligent Workflows helps ML engineers proactively rectify errors and ensure the reliability and robustness of AI model performance in production. This technology aims to transform the AI industry by addressing one of the most critical challenges faced by ML engineers today.
“A prominent concern voiced by our customers is the uncertainty surrounding where and how to detect issues during model failure before it is too late,” said Dr. Pavol Bielik, Chief Technology Officer and co-founder of LatticeFlow. “This is why we've engineered our model diagnostics tool, which guides machine learning engineers through a series of intelligent workflows to detect and access model blind spots, regardless of whether they use off-the-shelf AI models or custom architectures.”
Athena AI is one example of how LatticeFlow’s approach to eliminating model blind spots has helped its enterprise customers to scale the deployment of trustworthy AI models with greater confidence.
LatticeFlow’s model diagnostics capabilities allowed Athena AI to plug in its custom models with ease and discover hidden systematic errors. Fixing these errors resulted in 23% increased accuracy for some challenging classes in one of Athena AI’s models.
“LatticeFlow’s workflows significantly expedited our process of checking annotation quality – yielding a 3x speed improvement,” Rahul Kota, AI Lead at Athena AI.
Enabling early elimination of blind spots in the AI development cycle accelerates model performance improvement and risk mitigation. This fosters a more responsible and trustworthy AI ecosystem for enterprise ML teams.
Edited by
Alex Passett